AI bias in marketing: simple steps to keep your data accurate
AI bias can skew marketing data, harming insights, customer trust, and ROI. Here are some tips to prevent it.
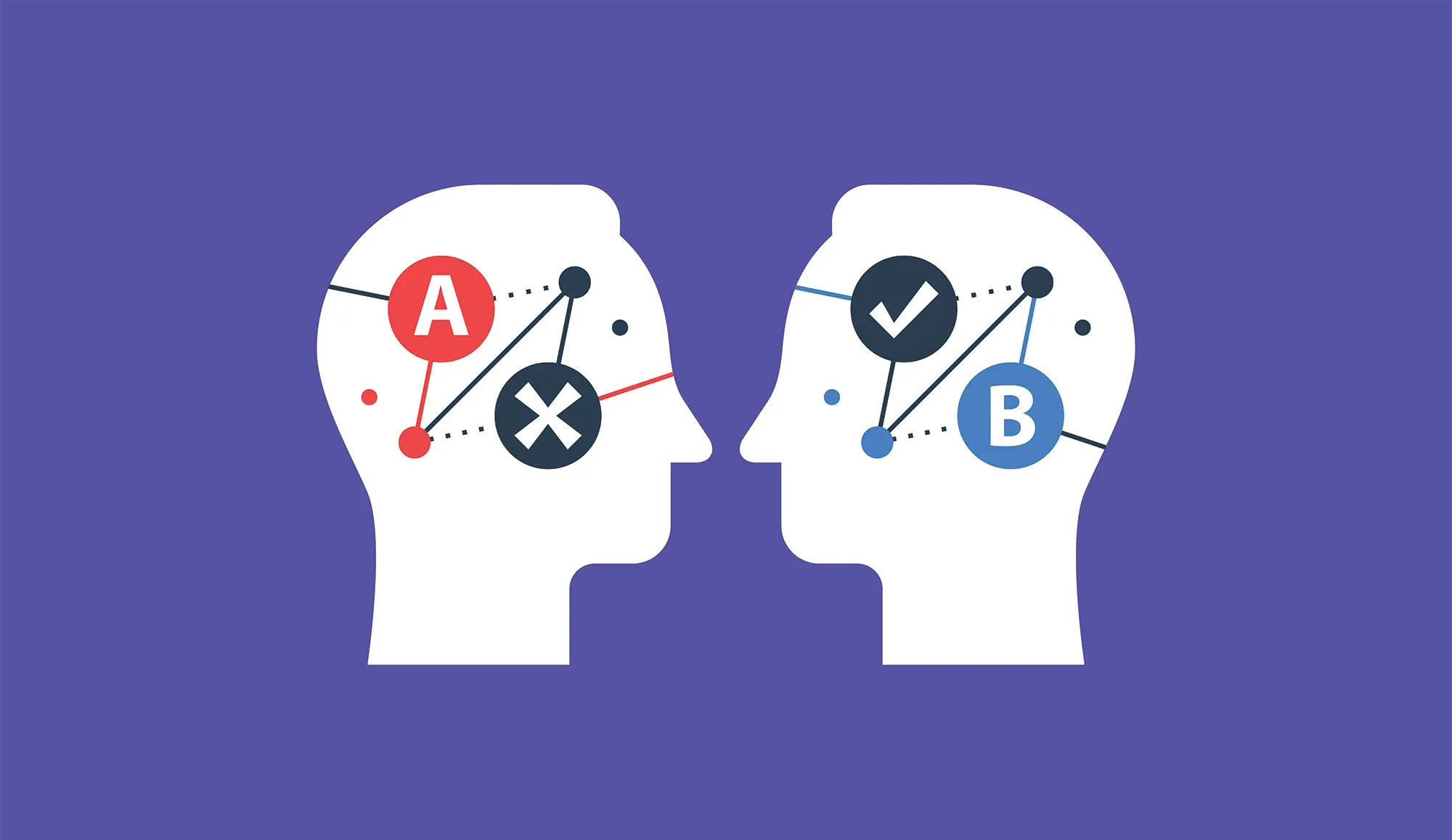
As artificial intelligence (AI) increasingly integrates into marketing, the risk of AI bias rises. When AI models are biased, they distort marketing data, which can harm campaign effectiveness and brand reputation.
AI bias in marketing is a growing concern, and data from McKinsey’s 2021 Global AI Survey illustrates the magnitude of this issue. The survey found that 40% of companies using AI had experienced unintended bias within their models, particularly impacting customer interactions and personalization efforts.
Additional data from the survey further shows 47% of executives who indicate that their organizations lacked sufficient tools to detect and address these biases, underscoring the urgent need for bias-detection strategies to prevent skewed customer insights and marketing missteps.
This article aims to take a closer look at AI bias risks and how marketers can protect their data.
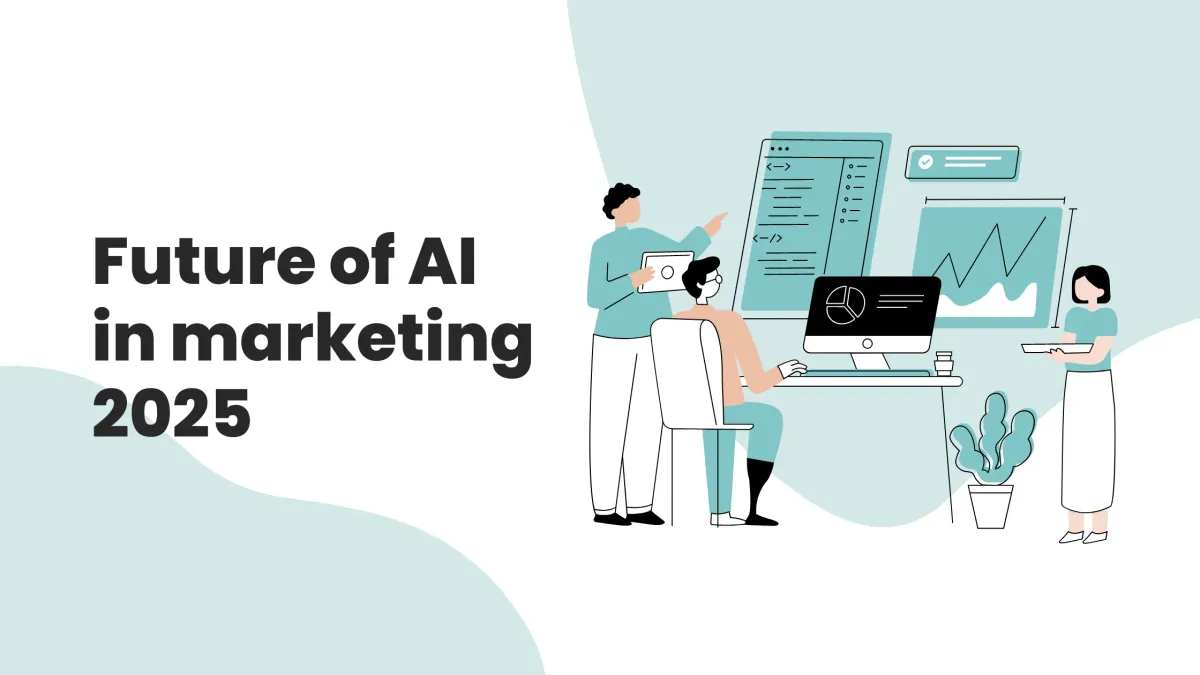
How AI bias can damage marketing data
When left unchecked, AI bias can cause specific problems for marketing data and insights:
- Distorted market segmentation - Bias can skew market segments, misdirecting campaigns and missing key audiences.
- Misguided product development - Biased data may lead to product decisions based on inaccurate preferences, missing the true needs of customers.
- Unfair competitive analysis - AI bias can produce distorted competitor insights, leading to flawed strategies.
- Inaccurate predictive modeling - Predictions skewed by bias lead to misguided forecasts, harming long-term planning.
- Loss of customer trust - If consumers perceive bias in interactions, they may lose trust in the brand, affecting loyalty and reputation.
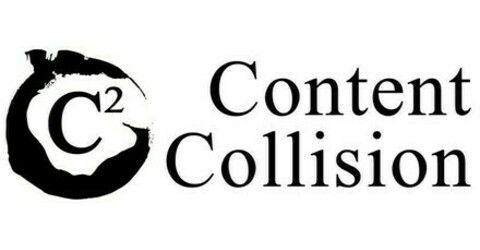
Tips and tools for marketers to manage AI bias
Marketers can take these actions to reduce AI bias and improve data accuracy:
1. Diversify training data
Ensure that AI training data reflects diverse customer segments to reduce bias. Tools to assist include:
- Google Dataset Search - Locates a wide range of datasets.
- Amazon SageMaker Ground Truth - Helps with data labeling and diversity.
- Figure Eight - Collects diverse data for machine learning.
2. Conduct Regular Audits
Regular audits help identify and address AI bias:
- IBM AI Fairness 360 - Monitors bias in AI models.
- Fairness Indicators by TensorFlow - Checks for bias in predictions.
- Google Model Cards - Documents model performance across demographics.
3. Emphasize Transparency
Transparency builds trust by clarifying AI’s role in decision-making. Tools for transparency include:
- Explainable AI by Google Cloud - Offers insights into AI decisions.
- Microsoft’s InterpretML - Explains machine learning models.
- Hugging Face Model Hub - Shares model data, enhancing transparency.
4. Collaborate with Experts
Working with ethics experts provides insights into bias prevention.
5. Stay Informed
Keeping up with AI developments helps marketers manage bias more effectively. Learning resources include:
- Coursera - Offers courses on AI ethics and bias reduction.
- KDnuggets - Provides articles on AI trends and ethical practices.
- DeepL AI Resources - Updates on AI advancements and tools for bias management.
By understanding the risks and implementing proactive measures—such as diversifying training data, conducting regular audits, ensuring transparency, and staying informed on new AI developments—marketers can protect the integrity of their data and their brand’s reputation.
ContentGrow is a managed talent network for brands and publishers to work with high-quality freelance writers and journalists worldwide. Sign up to get started or book a discovery call to learn more.
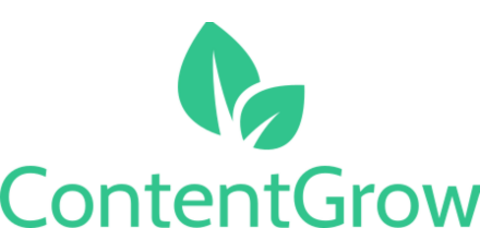